No. 11 - Minh H. Pham
Minh H. Pham: Axial Movements in Older Adults and Patients with Parkinson’s Disease – Algorithm Development and Validation with Inertial Measurement Units Data
To appear soon, 2019
Commission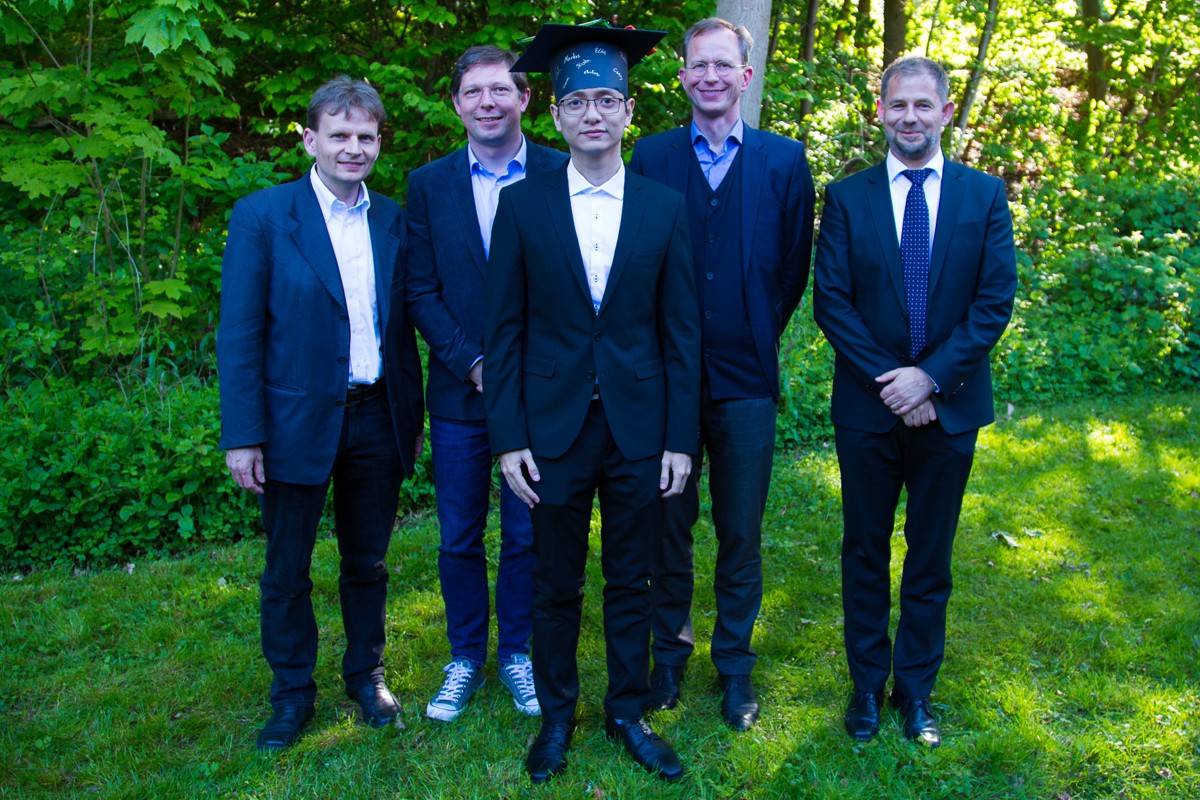
- Prof. Dr.-Ing. Gerhard Schmidt
(first reviewer) - Prof. Dr. med. Walter Maetzler
(second reviewer) - Prof. Dr.-Ing. Andreas Bahr
(examiner) - Prof. Dr.-Ing. Michael Höft
(head of the examination board)
Abstract
Movements that deviate from physiological performance are associated with many disabilities and reduce the ability to perform daily activities. These impaired movements are associated with e.g. aging and neurodegenerative diseases. An objective and quantitative evaluation of these impaired movements is of high clinical relevance, for both patients and the professional medical team that treats the patient. Moreover, assessment in the usual environment of the affected persons may be superior to assessments performed in the clinic and doctor’s practice, because the latter environments may lead to artificial results and can only be performed at certain time points.
The dynamic development of mobile technological devices has led to a new era of assessment in the medical field. Assessment of movements, especially axial (i.e. close to body center / trunk) movements are especially interesting for this development as sensors that detect movements accurately – e.g. accelerometers, gyroscopes and magnetometers – are especially far developed, reasonably priced and easily to integrate in mobile technology. However, there is a substantial lack of useful and, particularly, of validated algorithms for sensors and inertial measurement units that detect quantity and quality of specific movements in vulnerable cohorts. This work contributes to this area to such an extent, as it presents and discusses three algorithms that detect and evaluate specific movements detected with an inertial measurement unit (IMU) worn on the lower back by older adults and patients with Parkinson’s disease (PD). This work includes the evaluation of data from the supervised and unsupervised environment, and the validation of each algorithm.